How to fly to safety without overpaying for the ticket
Published in Economics and Business Review, Volume 9(2), 2023
Joint work with Przemysław Grobelny
Abstract: For most active investors treasury bonds (govs) provide di-versification and thus reduce the risk of a portfolio. These features of govs become particularly desirable in times of elevated risk which materialize in the form of the flight-to-safety (FTS) phenomenon. The FTS for govs provides a shel-ter during market turbulence and is exceptionally beneficial for portfolio drawdown risk reduction. However, what if the unsatisfactory expected return from treasuries discourages higher bonds allocations? This research proposes a solution to this problem with Deep Target Volatility Equity-Bond Allocation (DTVEBA) that dynamically allocate portfolios between equity and treasuries. The strategy is driven by a state-of-the-art recurrent neural network (RNN) that pre-dicts next-day market volatility. An analysis conducted over a twelve year out-of-sample period found that with DTVEBA an investor may reduce treasury allocation by two (three) times to get the same Sharpe (Calmar) ratio and overper-forms the S&P500 index by 43% (115%).
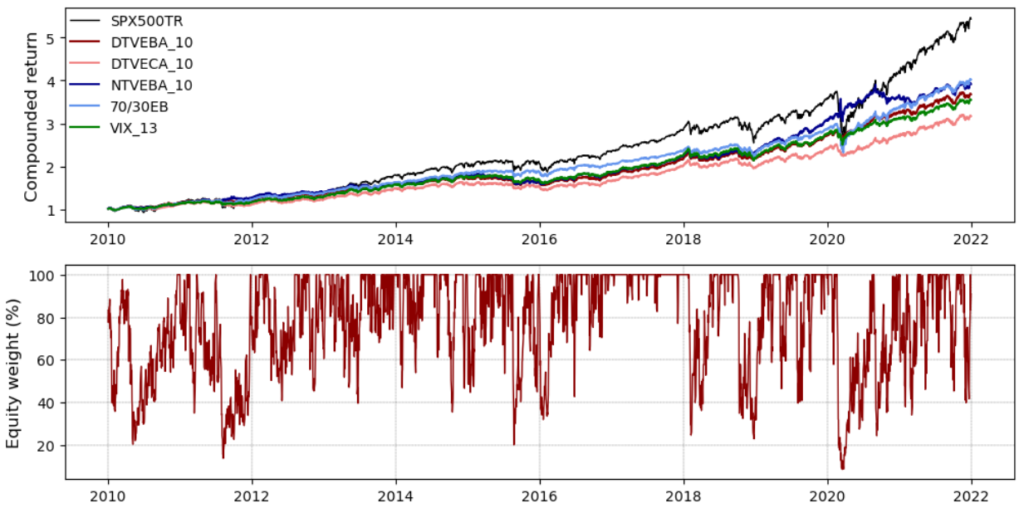
False Safe Haven Assets: Evidence From the Target Volatility Strategy Based on Recurrent Neural Network
Published in Research in International Business and Finance”, 101610, 2022
Joint work with Barbara Będowska-Sójka, Przemysław Grobelny, and Katarzyna Perez
Abstract: This paper examines which safe haven assets should be used when improving out-of-sample portfolio performance. We define a market state with recurrent neural network (RNN) volatility predictions and construct an investment strategy that dynamically combines equity, cash, and safe havens. The equity is allocated by targeting the volatility, and investing in safe havens depends on the predicted market state. We consider the S&P500 index with 13 safe haven assets, such as long-term government bonds, commodities, gold, and other precious metals. Other indices, NIKKEI225, NIFTY50, and STOXX50, are examined for robustness. With analysis conducted over a 20-year sample period, we find that RNN delivers sound predictions to construct the volatility targeting strategy. Among considered assets, only long-term Treasury bonds act as a safe haven and improve the strategy performance. Other considered assets have no such potential. Our findings are relevant to portfolio managers and investors actively managing portfolio risk.
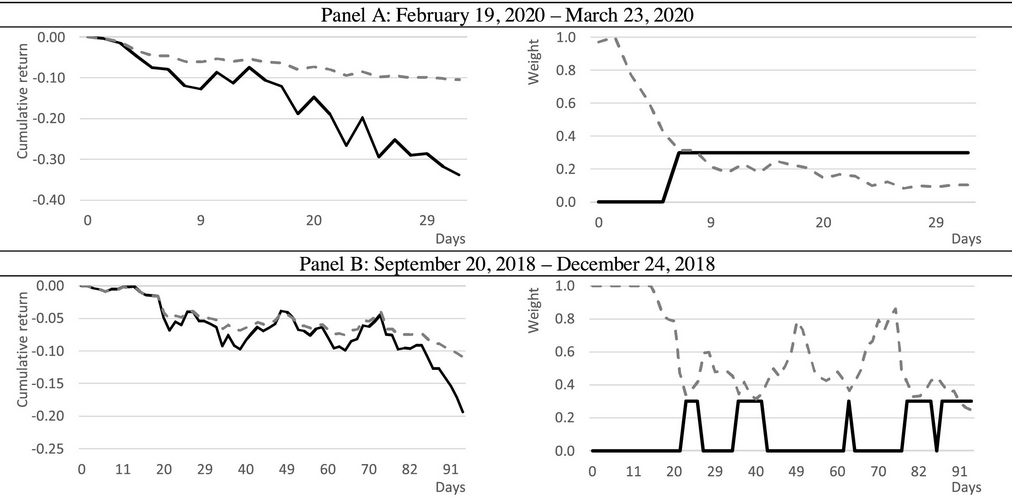
Building portfolios based on machine learning predictions
Published in Economic Research-Ekonomska Istraživanja, Volume 35, 2022
Joint work with Katarzyna Perez
Abstract: This paper demonstrates that portfolio optimization techniques represented by Markowitz mean-variance and Hierarchical Risk Parity (HRP) optimizers increase the risk-adjusted return of portfolios built with stocks preselected with a machine learning tool. We apply the random forest method to predict the cross-section of expected excess returns and choose n stocks with the highest monthly predictions. We compare three different techniques—mean-variance, HRP, and 1/N— for portfolio weight creation using returns of stocks from the S&P500 and STOXX600 for robustness. The out-of-sample results show that both mean-variance and HRP optimizers outperform the 1/N rule. This conclusion is in the opposition to a common criticism of optimizers’ efficiency and presents a new light on their potential practical usage.
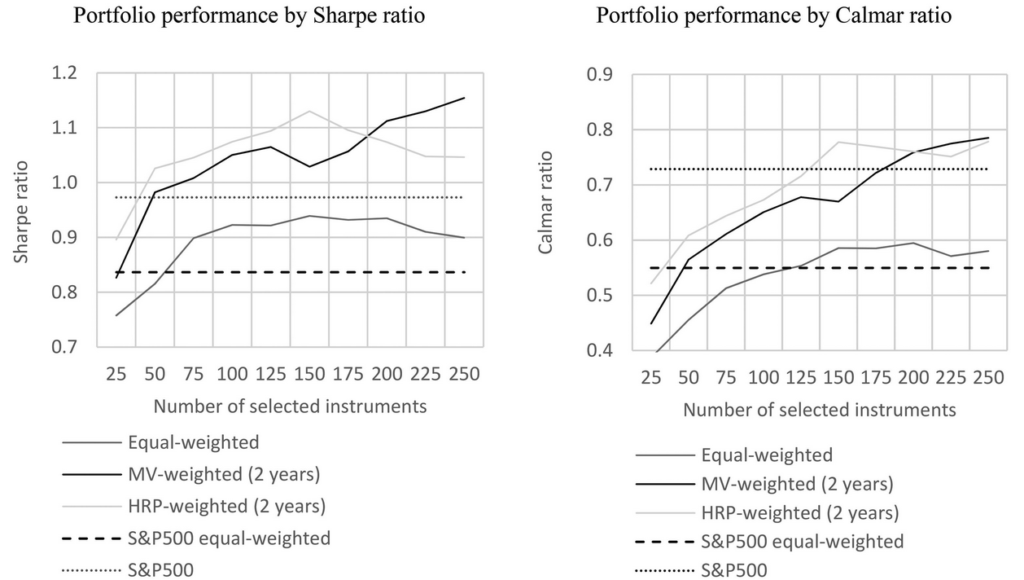
How to survive a pandemic: The corporate resiliency of travel and leisure companies to the COVID-19 outbreak
Published in Tourism Management, 84: 104281, 2021
Joint work with Katarzyna Perez, Ender Demir, and Adam Zaremba
Abstract: What protects travel and leisure companies from a global pandemic, such as COVID-19? To answer this question, we investigate data on over 1200 travel and leisure companies in 52 countries. We consider 80 characteristics, such as company financial ratios, macroeconomic variables, and government policy responses. Using regressions and machine learning tools, we demonstrate that firms with low valuations, limited leverage, and high investments have been more immune to the pandemic-induced crash. We also find a beneficial effect of stringent containment and closure policies. Finally, our results indicate that countries with less individualism may be better positioned to cope with the pandemic. Our findings have implications for regulatory bodies, managers, and investors concerning future pandemic outbreaks.
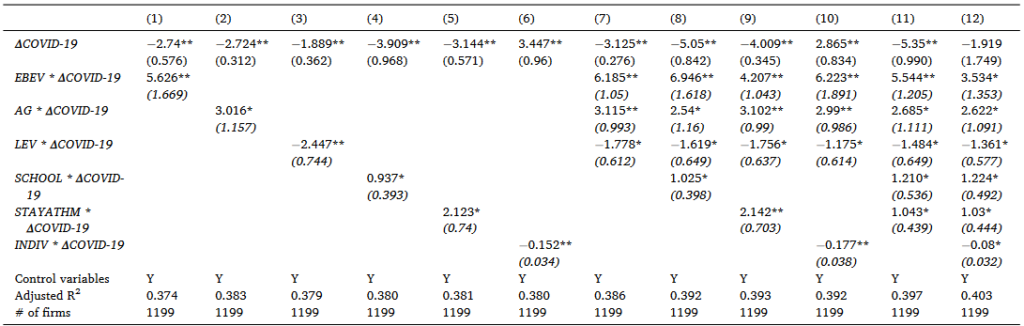
Uczenie maszynowe w budowie portfela inwestycyjnego
Chapter in Innowacje finansowe w gospodarce 4.0 (ss. 159–178), published by Wydawnictwo Uniwersytetu Ekonomicznego w Poznaniu, 2021
Joint work with Przemysław Grobelny and Mateusz Piotrowski